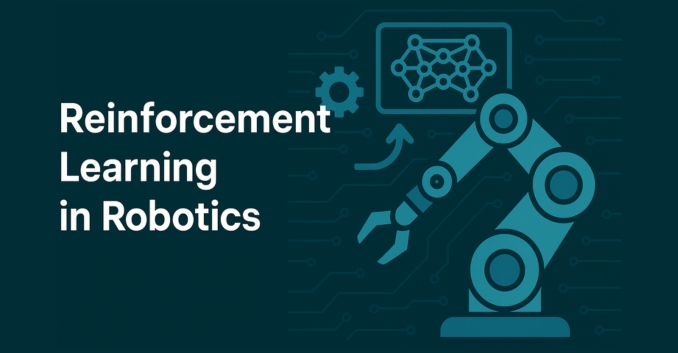
Reinforcement Learning: How AI is Shaping the Future of Robotics
Reinforcement Learning (RL) is a vigorous division of artificial intelligence that allows several systems to learn on their own with the help of interacting with the environment in which it is based. So unlike other forms of learning, RL is based on trial and error type learning where the main motive is to improve the actions for better gains. This suits it particularly for robotics since real-time decisions and adaptability are desirable technology characteristics. Therefore, RL, which models how humans learn from feedback, is revolutionizing the possibilities of robots in all fields.
Key Concepts in Reinforcement Learning
Reinforcement Learning operates on four key components: Agents, environments, rewards, and policies.
- Agent: The decision-maker (e.g. Robot)
- Environment: The environment within which the agent makes decisions.
- Reward: Reply suggesting the effectiveness of an action.
- Policy: The plan by which the agent gets his work done.
Common RL techniques include Q-learning, where agents acquire value-based, or more resourceful, actions; Deep Q Learning (DQL), which works on neural networks for challenging environments; and Policy Gradient Methods, which directly search for better actions. Consequently, these tools allow robots to perform more complicated tasks.
Applications of RL in Robotics
1. Autonomous Navigation
Reinforcement Learning is central in training robots to create and move around in an environment map. Such examples include self-driving car training to recognize traffic flow and drones to adapt to the best flight plan. The RL algorithms ensure safety and efficiency, allowing these robots to pre-program and respond to unexpected situations.
2. Industrial Automation
In the manufacturing process, RL is useful in assisting robots with aspects of the production process, such as assembly, inspection, and product movement. Temporary changes within parts or improvements in the paths of automated vehicles are acquired, decreasing expenses and increasing efficiency.
3. Humanoid Robots
Virtual reality with RL revolutionizes humanoid robots to re-create human behavior and actions. They include liberties such as walking, gripping objects, and engaging with humans, all now possible in RL. One example is OpenAI’s Dactyl system, designed to train a robotic hand to solve a Rubik’s Cube with unmatched precision and flexibility.
Challenges in Using RL for Robotics
Despite its promise, RL faces challenges in robotics:
- High Computational Costs: RL typically needs vast simulations and real-life testing, which are computationally intensive steps.
- Balancing Exploration and Exploitation: It is still challenging to determine what proportion of new actions and extended strategies should be employed.
- Safety Concerns: Trial and error is somewhat dangerous in physical robots since unsafe actions might cause harm or destruction.
Future Trends in RL for Robotics
The future of RL in robotics looks bright with emerging trends:
- Multi-agent RL: Cobots are designed to perform the tasks collectively for better productivity in areas such as logistics.
- Integration with Computer Vision: Integrating RL with more sophisticated vision systems will allow robots to make better decisions based on their context.
- Sim-to-Real Transfer: Training robots in simulations and then transitioning them to actually dealing with real-life situations will supplement the theory.
The Bottom Line
Indeed, robotics is among the fields where reinforcement learning can be a real game-changer since the more advanced robots are proposed to the market, the better and more complex tasks they can address. However, challenges, such as computational cost and safety, must also be considered to move forward. It is crucial to bring together AI researchers and roboticists to utilize RL and take robotics forward correctly. Altogether, all these innovations guarantee a revolutionary age for artificial intelligence and robotics.
Recent News
- Key Elements to Look for in a Machine Learning Services Provider in the USA
- Reinforcement Learning: How AI is Shaping the Future of Robotics
- How Artificial Intelligence is Transforming Personalized E-Learning
- Why the USA Leads in Artificial Intelligence and Machine Learning?
- Artificial Intelligence and Machine Learning in the USA: Trends & Innovations